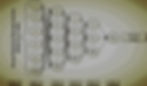
Deep Learning is making miracles in almost all areas of predictive science. I applied the same to predict how micro-machines (proteins) in our body interact to produce various biological or fatal (cancers : ( ) reactions.
Motivation: Protein forms specific molecular complexes and specificity of its interaction is highly essential for discovering and analyzing functional roles. Protein complexes are common in vital processes such as catalysis, immunity and cellular metabolic regulations. Protein-Protein interactions are found to form homo or hetero complexes. There are numerous methods to study protein-protein interactions, includes in vitro, in vivo and computational approaches. In vitro and in vivo methods promise higher reliability and accuracy on results but lag in terms of speed, time and high throughput. Computational approaches are far better than in vitro and in vivo methods in terms of cost, time, and efficiency. Recently there is huge acceleration in terms of uncovering novel protein-protein interaction due to the development of large-scale high-throughput experiments. In computational approaches, the domain-domain interaction based methods majorly emphasize on protein domain features which can more effectively predict PPIs. In this thesis, an integrative domain-based method is proposed for predicting PPIs using Deep Neural Network (DNN).
Results:
Various relevant features of proteins were exploited from the Database of Interacting Proteins (DIP) and Kansas University Proteomics Service (KUPS). In this article, domain-frequency based method is proposed by using DNN. When performed on the PPI data of Saccharomyces cerevisiae, the method achieved a very promising prediction result. An independent data set of 14,200 yeast PPIs was used to evaluate this prediction model and the prediction accuracy, precision, sensitivity and specificity obtained was 92.67%, 98.31%,86.85% and 98.51% respectively. Similar method was applied to PPI datasets of different size of Escherichia coli, Drosophila melanogaster, Homo sapiens and Caenorhabditis elegans, where accuracy was found to be 97.01%, 90.85%, 94.47% and 88.91% respectively. The performance of this method is superior to those of the existing domain-based machine learning methods, so this approach can be useful for predicting novel protein-protein interactions with high sensitivity and specificity. A web-based server was implemented on various organisms such as Saccharomyces cerevisiae, Caenorhabditis elegans, Drosophila melanogaster, Homo sapiens and Escherichia coli. The information related to datasets, prediction software, and supplementary files used in this paper are available on server interface - http://bioserver.iiita.ac.in/deepinteract.
This paper claims the highest accuracy achieved till time for protein-protein interaction prediction.
Research paper got published in Current Bioinformatics with impact factor - 0.99.
Research paper :
Author(s): Sunil Patel, Rashmi Tripathi, Vandana Kumari and Pritish Kumar Varadwaj
Affiliation: Department of Bioinformatics, Indian Institute of Information Technology Allahabad, Uttar Pradesh, India.